Unraveling the Intricacies of Anomaly Detection in the Age of AI
- Syed Zaid
- Jan 26, 2024
- 2 min read
Updated: Feb 9, 2024
In today's data-driven world, where vast amounts of information flow through digital networks, anomaly detection has become a critical aspect of maintaining the integrity and security of systems. Anomaly detection refers to the identification of patterns or instances that deviate significantly from the norm within a dataset. In the realm of artificial intelligence (AI), the deployment of sophisticated algorithms for anomaly detection has revolutionized the way we safeguard against threats, predict future trends, and ensure the smooth functioning of complex systems.
The Essence of Anomaly Detection
Anomaly detection is akin to finding a needle in a haystack, where the needle represents unusual patterns or outliers within a sea of data. Traditional methods often fall short in identifying subtle deviations, making anomaly detection a perfect candidate for AI intervention. Machine learning models, particularly those based on unsupervised learning, excel in recognizing anomalies by learning the normal patterns within data and flagging anything that diverges significantly.
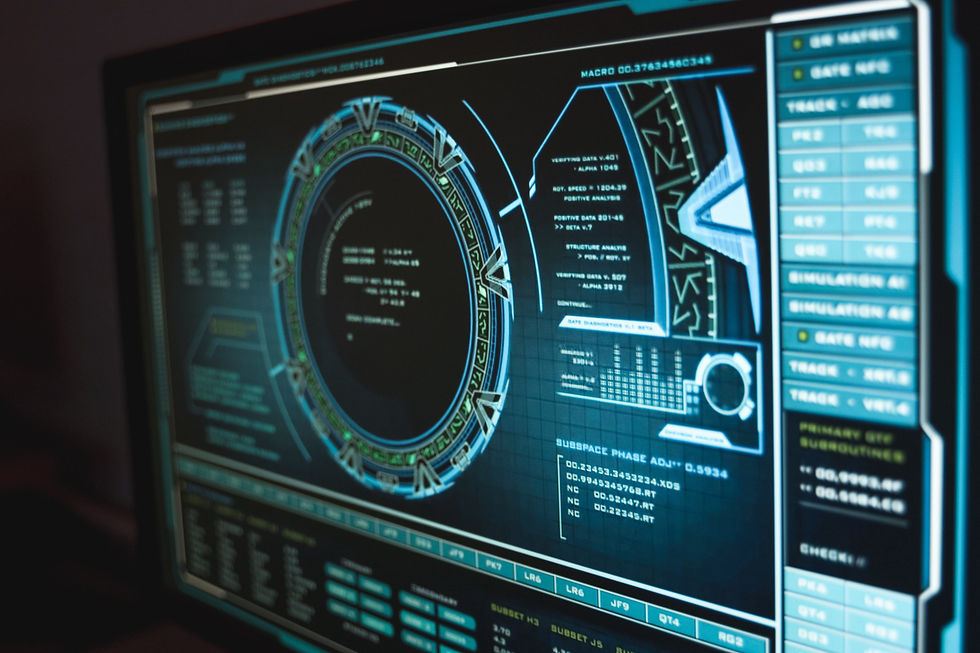
Applications Across Industries
The applications of anomaly detection are as diverse as the industries it serves. In cybersecurity, anomaly detection plays a crucial role in identifying unusual network behavior, potential security breaches, and abnormal user activities. Financial institutions leverage anomaly detection to spot fraudulent transactions or detect irregularities in trading patterns. In healthcare, it aids in the early detection of diseases by identifying unusual patient symptoms or deviations from normal physiological patterns.
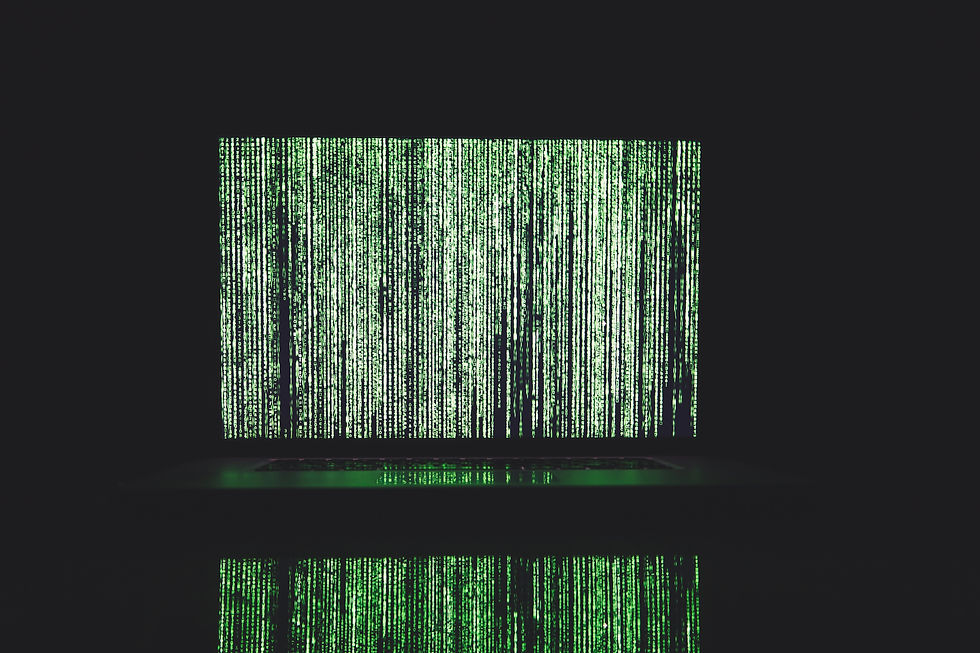
The Role of AI in Anomaly Detection
AI algorithms, including but not limited to machine learning and deep learning models, are the backbone of modern anomaly detection systems. These algorithms can sift through massive datasets, adapt to changing patterns, and provide real-time insights. For instance, in fraud detection, AI models can analyze transaction data and identify anomalies that might indicate fraudulent activities, thereby preventing financial losses.

Challenges and Solutions
Despite its power, anomaly detection is not without challenges. One significant hurdle is the potential for false positives, where normal variations are incorrectly flagged as anomalies. Striking the right balance between sensitivity and specificity is an ongoing challenge that researchers and practitioners are actively addressing. Furthermore, as data sources become more diverse and complex, ensuring the scalability and adaptability of anomaly detection models remains paramount.
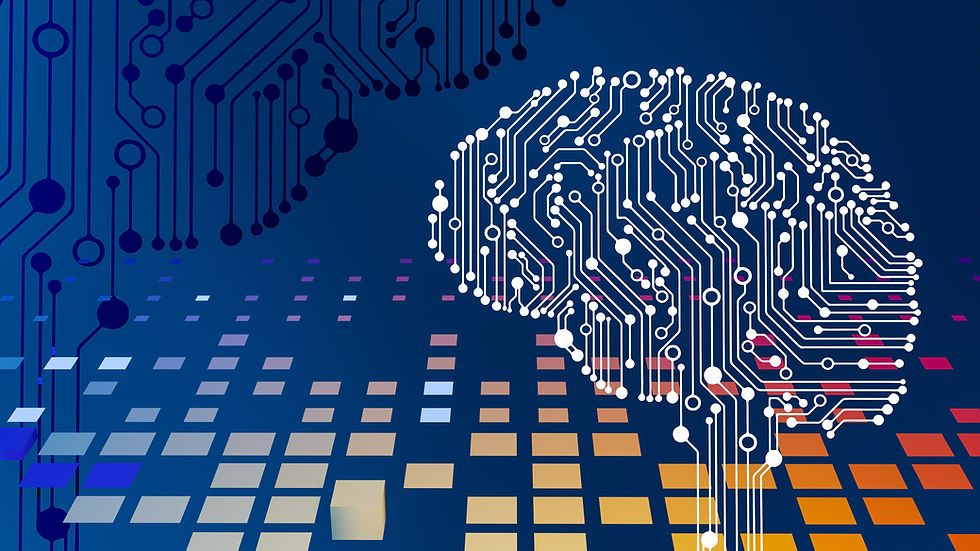
Future Frontiers
As technology continues to evolve, the future of anomaly detection holds exciting prospects. The integration of anomaly detection with other AI technologies, such as natural language processing and computer vision, is poised to provide more comprehensive insights. The rise of explainable AI is also crucial, as understanding why a system flagged a particular instance as anomalous is essential for trust and transparency.

In the intricate landscape of data analytics and artificial intelligence, anomaly detection stands as a sentinel, guarding against the unexpected and the malicious. Its applications span across industries, contributing to the robustness of cybersecurity, the efficiency of financial systems, and the early detection of health issues. As we delve deeper into the age of AI, the synergy between advanced algorithms and anomaly detection promises a future where anomalies are not just detected but thoroughly understood, setting the stage for a more secure and resilient digital world.
Comentarios